-
Address:
17888 67th Court North
Loxahatchee, FL
-
Mail us:
contact@wrightacademia.org
- submit manuscript
Table of content
Review Article |
Open Access |
Volume 1 | Issue 1 |
Stratification of Operative Time in Robotic-Assisted Laparoscopic Prostatectomy Assisted by Robot: Comparison between 100 First Cases by a Single Surgeon
Matheus NM Reis1 , Clayton VM Soares2, Jairo AG Galvis3, Diego DW e Sousa4, Eliney Ferreira Faria5 and Carlos VdM Maciel5
1Fellowship Robotic Surgery, Hospital Felicio Rocho de Minas Gerais, Brazil
2Urologist, Hospital Felicio Rocho de Minas Gerais, Brazil
3Resident Physician, Urology Department, Hospital Felício Rocho de Minas Gerais, Brazil
4Medical Student, Faculdade de Ciências Médicas de Minas Gerais, Brazil
5Urologist Preceptor, Hospital Felício Rocho, Brazil
*Corresponding author: Matheus NM Reis, Fellowship Robotic Surgery, Hospital Felicio Rocho de Minas Gerais, 9530 Contorno Avenue, Belo Horizonte 30110-934, Brazil
Citation: Reis MNM, Soares CVM, Galvis JAG, Sousa DDW, Faria EF, et al. (2021) Stratification of Operative Time in Robotic-Assisted Laparoscopic Prostatectomy Assisted by Robot: Comparison between 100 First Cases by a Single Surgeon. J Surg Clin Rpts 1:001.
Copyright © Reis MNM.
Received: |
Accepted: |
Published: |
Introduction: Prostate cancer (PC) can be treated by surgical procedures in different approaches: Open (PR), laparoscopic radical prostatectomy (LRP) or robot-assisted laparoscopic prostatectomy (RALP). The RALP has proved to be a promising technique, with satisfactory functional and oncological results. This study aimed to analyze the learning curve of a surgeon in RALP.
Methods: This is a retrospective investigation with data obtained from the analysis of medical records of the first 100 patients undergoing RALP surgery from October 2018 to August 2019 at single institution in Brazil, by a single surgeon. We divided them into two groups according to the chronological surgery. The studied variables were surgical time divided in console, docking and undocking, robot preparation, anesthesic induction, wall closure. Blood loss, surgical margins, time of hospitalization were also measured.
Results: The total surgical time, anesthetic induction time, and robot preparation time were statistically different between the groups. Regarding the length of stay and postoperative factors, there was no significant difference.
Conclusion: The total surgical time decreases as the whole team's experience increases. The total surgical time was optimized mainly by improving the anesthetic induction and robot preparation times.
Introduction
Prostate cancer (PC) is the most prevalent tumor among men and the fifth in number of deaths worldwide. The incidence is approximately 1.28 million cases every year [1]. Brazil related 15576 deaths in 2018 and estimates 65840 new cases in 2020 [2].
PC has a multifactorial etiology which family history, especially in first-degree relatives, is associated with an increased risk of the disease. Age, obesity, and the afrodescendent ethnicity are some other risk factors described [3,4].
Therapeutic options of localized PC includes external radiotherapy, brachytherapy and radical prostatectomy, none of which showed superiority over the others in terms of overall survival [4,5]. The last one is considered the gold standard for localized or locally advanced disease in patients with life expectancy of more than 10 years [6,7].
Surgical treatment can be performed by three different techniques: Open radical prostatectomy (ORP), laparoscopic radical prostatectomy (LRP) or robot-assisted laparoscopic prostatectomy (RALP). A recent systematic review found no statistically significant difference between oncological and functional outcomes (urinary continence and sexual potency). In terms of hospitalization time and need for hemotransfusion, minimally invasive techniques showed better results [8].
The RALP shows promise as a technique presenting satisfactory functional and oncological results. Current literature has suggested that basic proficiency in robot-assisted laparoscopic surgery can be achieved with a relatively few number of cases, less than the learning curve of laparoscopic radical prostectomy, whose basic skill is achieved from 25 to 40 cases. However, the amount of cases needed to achieve mastery in RALP is unclear [9]. Three-dimensional (3D) vision, better ergonomics, image amplification, greater amplitude and degree of movement in the clamps are some of the additional benefits of robotic technology [10].
The greatest obstacle to the dissemination of the robotic platform in Brazil is the high costs, in addition to the presence of few training and improvement centers for surgeons.
The purpose of this study is to analyze the learning curve of a single surgeon, with extensive experience in laparoscopy, robot-assisted radical prostatectomy for the treatment of prostate cancer.
Materials and Methods
Study facilities
The research was done at the Felicio Rocho Hospital, a private tertiary care institution located in Belo Horizonte, Minas Gerais state in Brazil. With a capacity of approximately 300 rooms. An average of 30 robotic surgeries is performed per month, 20 of which are radical prostatectomies.
Study design and sample size
Retrospective study, with analysis of the first 100 RAPs performed by the same surgeon, with experience in laparoscopic surgery, between October 2017 and August 2019.
He has done a fellowship program in video laparoscopy and robotics in France at Institute Mutualiste Montsouris in 2007. The robotic program has started at Felicio Rocho Hospital in 2017 and since then the surgeon started to do the robotic surgeries. He has already done about 200 cases.
The surgeries occurred through transperitoneal access, with antegrade dissection, without previous ligation of the plexus of the dorsal vein of the penis. The urethrovesical anastomosis was performed with continuous suture, as well as the reconstruction of the posterior urethra, with approximation of this to the bladder neck, as described by Rocco.
The patients were divided into two groups, each with 50 participants, according to the chronological order of the surgical procedures.
Inclusion criteria
Patients with localized and locally advanced prostate cancer undergoing RALP at Felicio Rocho Hospital between October 2017 and August 2019. Localized prostate cancer corresponds to stages T1-T2N0M0 while the locally advanced cancer concerns stage T3-T4 or N1.
Exclusion criteria
There was no exclusion criteria, all the first 100 cases were included.
Information collected
Patients were analyzed in relation to the following preoperative data: Age, body mass index (BMI), prostate-specific antigen (PSA), Gleason score on biopsy, prostate volume obtained by transrectal ultrasound. Body mass index was divided in 5 different groups: 1 (18.5 to 24.9 kg/m2), 2 (25 to 29.9 kg/m2), 3 (30 to 34.9 kg/m2), 4 (35 to 39.9 kg/m2), 5 (> 40 kg/m2).
The variables collected from the intraoperative period were blood loss and surgical time. The surgical time was divided into anesthetic induction, preparation of the robot, docking and undocking, introduction of the trocars, console, removal of the specimen, and wall closure. The console time was not subdivided in the different steps of the surgery but was calculated from the beginning to the end. The blood loss was stored in a graduated bottle connected to the aspirator used during surgery. After the procedure, the nurse assessed and noted the debt.
The postoperative variables were Gleason score in the biopsy of the piece, time of hospitalization, presence of compromised surgical margin and pathological stage defined by the American Joint Committee on Cancer (AJCC) [11]. There was no postoperative follow-up regarding the evaluation of functional results such as urinary continence and sexual potency.
There are no cataloged data about oncological, functional and perioperative variables related to laparoscopic surgeries performed by the surgeon. However, the objective of our research is to evaluate the learning curve in robotic surgery, without comparing it with the laparoscopic technique.
The nursing team in the robotic room was responsible for write down the surgical times in a spreadsheet. The total surgical time was calculated from the patient's entrance into the room until he left after the surgery. In this interval, the robot preparation time, anesthetic induction, introduction of trocars, docking, console, undocking and abdominal wall closure times were included.
Data analysis
The statistical analysis was performed through the chi-squared test and the Fischer test for the categorical variables.
The quantitative data were tested for normality by the Kolmogorov-Smirnov test.
The comparison of normal data was done by T Student and for those not normal, the Mann-Whitney test was used.
In all tests, the adopted confidence index was 95% which was considered significant when p < 0.05.
The software used for the analyses was SPSS version 20.0.
Results
100 patients were evaluated, divided into two groups of 50 each, according to the chronological order of the procedure. The first half formed group 1 which patients were submitted to robot-assisted laparoscopic prostatectomy from October 2017 to November 2018, while the second formed group 2, which patients were submitted to robot-assisted laparoscopic prostatectomy from November 2018 to August 2019.
The analysis of the preoperative variables, showed that the median of total PSA of Group 1 was 10.13 and that of Group 2 was 6.33. There was a statistically significant difference between them, considering p < 0.05. The average age was similar between both, just like the BMI (Table 1 and Table 2).
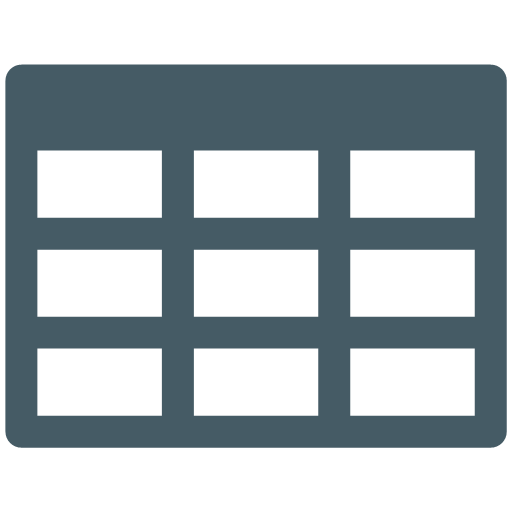
Table 2: Comparison Body Mass Index (BMI) between de groups, Table a-group 1, Table b-group 2. View Table 2
The prostate size calculated by transrectal ultrasound did not present a statistically significant difference between the groups although the number of positive fragments did. The proportion of the Gleason classification showed homogeneity between the groups when evaluating histopathological parameters of the disease (Table 3).
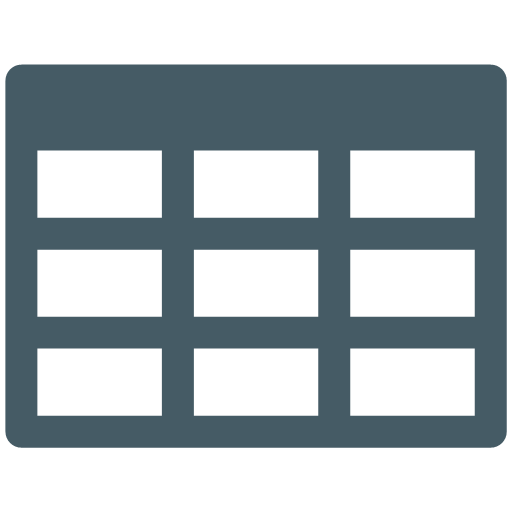
Table 3: Gleason distribution according to groups 1 and 2. View Table 3
Within the preoperative data, total surgical times, anesthetic induction and robot preparation presented statistically significant difference considering p < 0.05. All variables were higher in group 1 in relation to group 2. Surgery took, on average, 315 minutes for the first 50 patients and 290 minutes for the last ones. Initially, the average time of preparation of the robot was 12.3 minutes with decrease to 10.1 minutes in the second group. Bleeding volume, patient positioning time, console, undocking, and wall closure showed no difference between the study groups (Table 4).
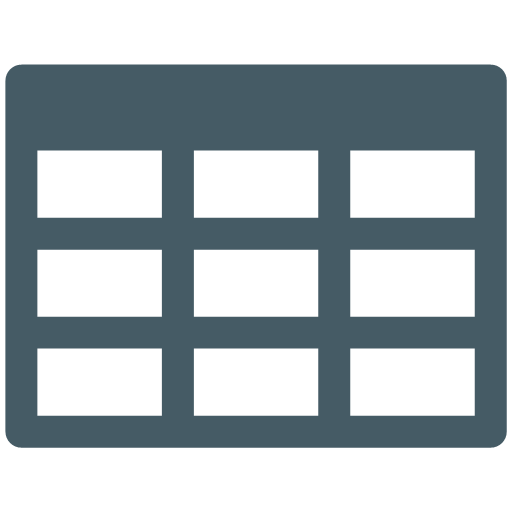
Table 4: Comparison of perioperative variables of Groups 1 (n = 50) and 2 (n = 50). View Table 4
The average time of anesthetic induction was 9.91 minutes in group 1 and 8.02 minutes in group 2. The average time of preparation of the robot was 33.64 minutes in the first group and 27.02 minutes in the second, the difference between then had statistical relevance (Table 4).
The volume of bleeding did not present significant difference between the groups, however, The measurement of intraoperative bleeding showed many methodological biases, for example urine aspirated at the bladder neck opening and serum for washing the cavity without the correct precision of this volume, so we chose to remove this variable from the comparative analysis.
There was no statistically significant difference between the groups when we compared postoperative data, either in relation to the time of hospitalization or to the study of pathological anatomy (Table 5). None of the patients needed blood transfusion, neither had any concomitant visceral lesions. No postoperative drain was used and no urinary fistula was suspected in the postoperative period.
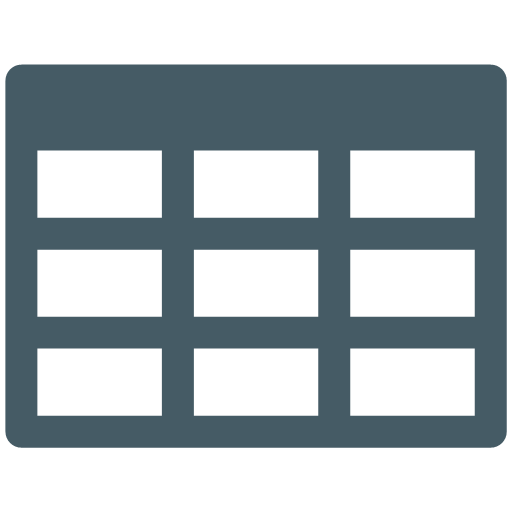
Table 5: Comparison of length of stay for groups 1 and 2. View Table 5
Positive surgical margins, presence of extra-prostatic disease, perineural invasion, T and N pathological stage, as well as seminal vesicle involvement, despite small alterations between the groups, showed no statistical difference between the populations studied (Table 6).
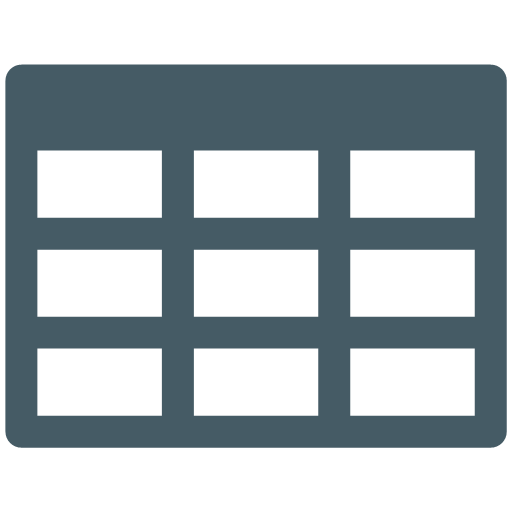
Table 6: Comparison of post-operative variables in relation to Groups 1 and 2. View Table 6
In group 1, 90% presented perineural invasion and 78% in group 2, also without statistical significance, with p = 0.102.
Tumor size and involved nodes (T and N stages) were also similar in groups 1 and 2, most of them with less advanced tumors.
Discussion
Different studies suggest that a surgeon with experience in laparoscopy, already familiar with the anatomy, would be able to acquire expertise in approximately 45 cases. There is no consensus in the literature about the benefits of experience in laparoscopy in reducing the robotic learning curve, however this is not an aim of our report [6,9,12].
The time between the patient entering and leaving the operating room showed a statistically significant difference when compared to both groups. For the first group it was 313.96 minutes, while for the second it was 288.56. Sierra, et al. published a significant reduction of that period in an analysis of 30 cases, subdivided between the first and last fifteen of the study, from 435 to 272 minutes, on average [13].
The same author also described a significant decrease in robot preparation and surgery times when comparing the first and last patients operated [13]. In our study, the robot preparation time showed a statistically significant difference between the groups, with a decrease from 34 to 27 minutes. The gain in experience of the nursing team, as well as the greater bonding with the surgeons and anesthesists, helps to reduce the period needed to prepare the platform. All nurses allocated to robotic surgeries receive frequent training offered by Intuitive® in order to improve the routine related to the surgical procedure.
Slusarenco, et al., published an average console time of 163 minutes for the first 49 cases, with significant improvement to 88 cases, up to 107 [14]. Badani, et al. showed an improvement in surgical time of about 20% when compared to the first 200 procedures performed with the last 200 in a series of more than 2000 patients [15]. Hashimoto, et al. reported a decrease in the average surgery time ranging from 202 to 498 minutes, to 130 to 195 minutes after 100 to 300 cases [16]. Jaffe, et al. found an abrupt decrease after the first 12 cases, with subsequent significant reduction after 189 procedures. Unlike the studies described above, when comparing the surgeon's time at the console, we found no alterations. It is possible that the number of procedures necessary for significant improvement of the variable has not yet reached a value close to those related to the previous studies [6].
The anesthetic induction time varies from 9.91 minutes for the first group to 8.02 for the second. These data suggest that the anesthesiologist's adaptation to the new technology generates benefits in relation to the decrease in time used.
The average total time of hospitalization was 2.1 days, with no statistically significant difference between the groups. Studies prior to 2010 showed longer periods, ranging from 4 to 7 days.
Jaffe, et al. in 2009, found no difference in the period of hospitalization of patients undergoing surgery along the learning curve. However, the average time was 5 days. [6] More recent publications such as Machado, et al. Slusarenco, et al., found no difference in the period of hospitalization during the learning curve [15,17]. With the familiarization and dissemination of the technique, there is improvement in the intraoperative procedure, with fewer complications, resulting in early hospital discharge [17].
An important variable in the analysis of the learning curve is the commitment of surgical margins. It is influenced by the experience of the surgeon, the technique employed and the characteristics of the tumor [17]. In our study, there was a decrease in the number of free margins in the second group relative to the first, however, there was no statistically significant difference.
Hashimoto, et al. published an overall rate of positive margins for the first fifty cases of 34% with a significant reduction for 19% in the 150 subsequent cases. It is important to highlight the high proportion of intermediate and high risk in the study [16,18]. Badani, et al. showed a lower percentage of compromised margins, being 7% in the first 200 and 4% in the last of a sample of 2766 prostate neoplasia carriers [15]. Slusarenco, et al. presented an average of 13.1% of compromised surgical margins without improvement after 145 cases of surgery. The hypothesis was raised that the learning curve had not yet reached its tipping point. Patel, et al. suggest that it is around 1500 surgeries [19]. It is possible that our casuistry has not yet reached the value suggested by Patel to have significant improvement in the free margins.
This study showed limitations such as retrospective analysis of data, lack of randomization, short postoperative follow-up that makes functional and oncological evaluation unfeasible. There was no division of the groups in relation to the tumor stage, which suggests alterations in the variables studied. The analysis of the learning curve is not uniform, with great variation among surgeons and takes into consideration the previous skills and experience of the professional who will perform the procedure.
Another limitation of the study is the number of procedures performed in order to assess improvements in surgical margins. Different published articles suggest a larger sample than the one carried out by us.
Conclusion
The surgical time decreases as the surgeon's experience increases as well as the connection and adjustment of the whole team involved in the surgical procedure. Analyzing the 100 robotic radical prostatectomies, we identified a significant improvement in the time of anesthetic induction and robot preparation with reflex in the reduction of the total surgical time. A greater number of cases are needed to assess improvement in the rates of involvement of surgical margins.
References
- Ferlay J, Soerjomataram I, Ervik M, et al. (2018) GLOBOCAN (2012): Cancer Incidence and Mortality Worldwide v1.0. IARC CancerBase Lyon: International Agency for Research on Cancer.
- Ministério da Saúde. Instituto Nacional de Câncer. Estimativas 2018: incidência de câncer no Brasil. Rio de Janeiro.
- Hemminki K (2012) Risco familiar e sobrevivência familiar no câncer de próstata. World J Urol 30: 143.
- Okano MTR (2014) Avaliação prospectiva de curva de aprendizado da prostatectomia radical laparoscópica assistida por robótica [tese]. São Paulo: Universidade de São Paulo.
- Mottet N, Bellmunt J, Bolla M, et al. (2017) EAU-ESTRO-SIOG guidelines on prostate cancer. Part 1: Screening, diagnosis, and local treatment with curative intent. Eur Urol 71: 618-629.
- Jaffe J, Castellucci S, Cathelineau X, et al. (2009) Robot-assisted laparoscopic prostatectomy: A single-institutions learning curve. Urology 73: 127-133.
- EAU Pocket Guidelines (2020) presented at the EAU Annual Congress Amsterdam 2020.
- Ilic D, Evans SM, Allan CA, et al. (2017) Laparoscopic and robotic-assisted vs. open radical prostatectomy for the treatment of localized prostate cancer. Cochrane Database Syst Rev 9: CD009625.
- Lavery HG, Samadi DB, Thaly R, et al. (2009) The advanced learning curve in robotic prostatectomy: A multi-institutional survey. J Robot Surg 3: 165-169.
- Orvieto MA, Patel VR (2009) Evolução da prostatectomia radical assistida por robô. Scand J Surg 98: 76-88.
- Edge SB, Compton CC (2010) The American Joint Committee on Cancer: The 7th edition of the AJCC cancer staging manual and the future of TNM. Ann Surg Oncol 17: 1471-1474.
- Ahlering TE, Skarecky D, Lee D, et al. (2003) Successful transfer of open surgical skills to a laparoscopic environment using a robotic interface: Initial experience with laparoscopic system. J Urol 170: 1738-1741.
- Moreno Sierra J, Núñez Mora C, Galante Romo MI, et al. (2008) Prostatectomía radical asistida por robot Da Vinci®: un año de experiencia en el hospital clínico San Carlos. Arch Esp Urol 61: 385-396.
- Slusarenco RI, Mikheev KV, Prostomolotov AO, et al. (2020) Analysis of Learning Curve in Robot-Assisted Radical Prostatectomy Performed by a Surgeon. Advances in Urology 2020: 9191830.
- Badani KK, Kaul S, Menon M (2007) Evolution of robotic radical prostatectomy: Assessment after 2766 procedures. Cancer 110: 1951-1958.
- Hashimoto T, Yoshioka K, Gondo T, et al. (2013) Learning Curve and Perioperative Outcomes of Robot-Assisted Radical Prostatectomy in 200 Initial Japanese Cases by a Single Surgeon. Journal of Endourology. 27: 1218-1223.
- Marcos TM, Ibrahim MA, Mauricio R, et al. (2016) Robotic-assisted radical prostatectomy learning curve for experienced laparoscopic surgeons: does it really exist?. Int Braz J Urol 42: 83-89.
- Ou YC, Yang CR, Wang J, et al. (2010) Robotic-assisted laparoscopic radical prostatectomy: Learning curve of first 100 cases. Int J Urol 17: 635-640.
- Patel VR, Tully AS, Holmes R, et al. (2005) Robotic radical prostatectomy in the community setting: The learning curve and beyond: Initial 200 cases. J Urol 174: 269-272.
INDEXING
PARTNERS

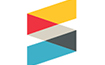
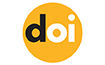
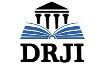
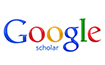
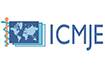


Table 1: Comparison of preoperative variables according to groups 1 (n = 50) and 2 (n = 50). View Table 1